일 | 월 | 화 | 수 | 목 | 금 | 토 |
---|---|---|---|---|---|---|
1 | 2 | 3 | 4 | 5 | ||
6 | 7 | 8 | 9 | 10 | 11 | 12 |
13 | 14 | 15 | 16 | 17 | 18 | 19 |
20 | 21 | 22 | 23 | 24 | 25 | 26 |
27 | 28 | 29 | 30 |
- deep learning #machine learning #history
- momentum #adagrad #adam #early stopping #data augmentation #dropout #label smoothing #batch normalization
- IOU
- objectdetection
- flops
- 부트캠프
- AI tech
- confusion matrix
- generalization #overfitting # bootstrapping #bagging #boosting #stochastic gradient desent #mini-batch gradient descent #batch gradient descent
- recall
- neural networks #linear #nonlinear #perceptrons
- map
- 1x1 convolution
- CNN #padding #stride #parameters
- FPS
- 네이버
- Optimization
- evaluation
- precision
- 일상
- 대학원생
- Today
- Total
목록네이버 부스트 캠프 (12)
공부 일기장
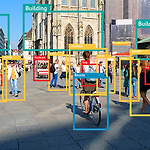
object detecion의 개념, 적용분야, history, evaluation, libarary에 관해 간략히 정리한 글입니다. Object Detection 객체의 영역과 클래스를 예측하는 Task 자율주행과 OCR, 의료와 같은 분야에서 많이 사용된다. History object detecion의 대략적인 history는 아래 그림과 같다. Evaluation 1. performance mAP (mean average precision) 각 클래스당 AP의 평균 confusion matrix Precision Recall PR Curve confidence 기준으로 내림차순하고 누적 TP,FP를 기준으로 precision, recall 를 계산한 표로 작성한 그래프 해당 그래프는 클래스 하나의 ..
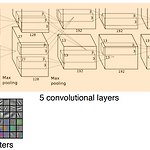
ILSVRC 에서 해마다 우수한 성능을 보였던 모델들의 핵심만 리뷰 모델이 발전해가면서 네트워크의 depth는 점점 깊어지면서 parameters는 점점 줄어든다. AlexNet 11x11 Input 파라미터 숫자 관점에서는 좋지 않은 선택 해당 필터는 receptive field는 넓어질 수 있지만 상대적으로 더 많은 파라미터가 필요 8-layers 5 conv + 3 dense Key ideas ReLU(Rectified Linear Unit) activation overcom the vanishing gradient problem good generalization GPI implementations(2 GPUs) Data augmentation Dropout VGGNet 3 x 3 convolut..
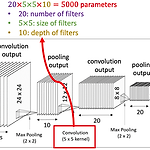
CNN [필수 개념] filter & channel stride padding pooling CNN의 기초적인 형태 가장 중요한 건 파라미터 수를 계산할 줄 알아한다. layer별로 또는 전체의 파라미터가 어느정도인지 감을 가지고 있는 것이 매우 중요 최근에 FC layer를 최대한 줄이는 추세임 (파라미터를 실제로 손으로 계산해보는 연습 또한 필요함) Stride Padding Parmeters Calculate stride 1, padding 1인 경우 다음의 파라미터 수는 (3*3*128)*64 = 73,728 1 X 1 Convolution 영역을 보지는 않고 한 pixel만을 본다. 그럼에도 사용하는 이유는? dimension reduction increasing depth reduce numb..
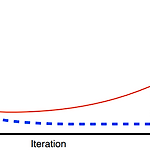
용어들에 대해서 확실하게 알고 넘어가보자 [관련 용어] Generalization 일반화 성능? - generalization gap이 작다 Under-fitting vs Over-fitting Cross-validation Bias-variance trade-off variance : 비슷한 입력들을 넣었을 때 출력 또한 일관적인지 bias : 입력들이 true target의 근처에 모여있는지 cost = bias + variance + noise trade-off 관계 Bootstrapping 여러 sampling data를 통해 여러 모델을 만드는 것 Bagging and boosting Bagging 학습데이터를 여러개를 만들어서(bootstrapping) 여러모델을 학습시키고 결과값을 평균 N개..